Chapter 6
A game changer for misinformation: The rise of generative AI
Lead authors: Victor Galaz, Stockholm Resilience Centre, Stefan Daume, Stockholm Resilience Centre, and Arvid Marklund, Stockholm Resilience Centre.
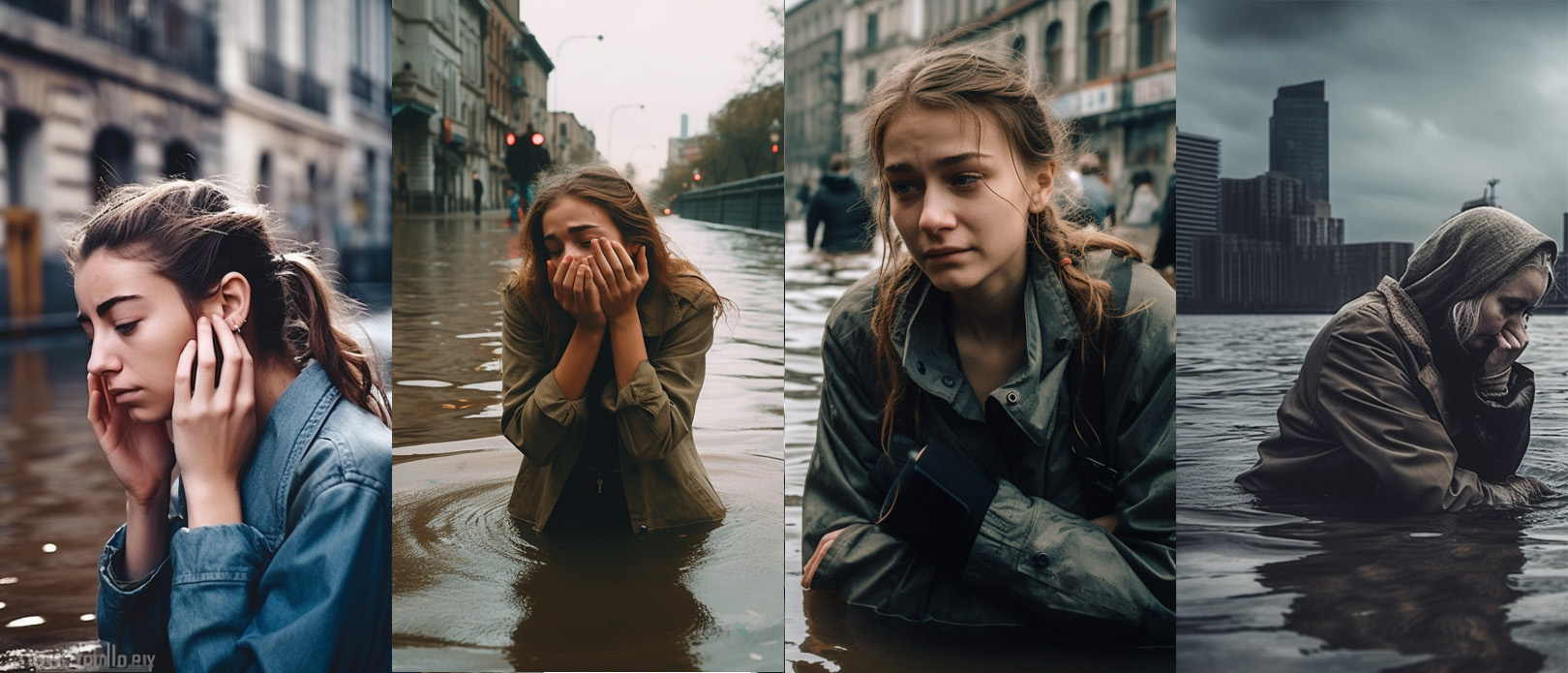
Generative AI images created by Diego Galafassi using four different image generators. The prompt was "A crying person in a flooded city due to climate change".
New generative AI tools make it increasingly easy to produce sophisticated texts, images and videos that are basically indistinguishable from human-generated content. These technological advances in combination with the amplification properties of digital platforms pose tremendous risks of accelerated automated climate mis- and disinformation.
The year 2023 will be mentioned in history books as the point in time when advances in artificial intelligence became everyday news – everywhere. The decision by OpenAI to offer the general public access to their deep learning-based Generative Pre-trained Transformer (GPT) model opened up a floodgate of experimentation by journalists, designers, developers, teachers, researchers and artists.
Generative AI systems such as these have the ability to produce highly realistic synthetic text, images, video and audio – including fictional stories, poems, and programming code – with little to no human intervention. The combination of increased accessibility, sophistication and capabilities for persuasion may very well supercharge the dynamics of climate mis- and disinformation.
Accessibility
Accessibility refers to the fact that generative AI tools that produce highly realistic synthetic content, that is not necessarily accurate, are rapidly becoming easily available.
While the most capable models remain either private or behind monitorable application programming interfaces (APIs), some advanced models are publicly accessible, either in open code repositories or through public APIs including OpenAI, Google, Microsoft and Hugging Face (Table). In addition, the open source community has quickly managed to create much smaller versions of large language models that are almost equally powerful, and can be run on laptops or even phones (Dickson, 2023). All of these tools can, in principle, be prompted to deliberately generate false information, both in the form of text (McGuffie and Newhouse 2020; Buchanan et al. 2021) and photo-realistic but fake images (Mansimov et al., 2016; Goldstein et al., 2023).
Table: Selection of generative language models released between 2018 and 2023. Language models are accelerating in parameter size, utilize growing training datasets, support multiple languages, and have the capacity to generate both text as well as images in response to text ‘prompts’. (1). Estimated. The report does not specify the number of parameters or training tokens. (2). For DALL-E the training set consists of text/image pairs. Table compiled by Stefan Daume.
A simple prompt in GPT-3, for example (“write a tweet expressing climate denying opinions in response to the Australia bushfires”), results in short and snappy climate denial pieces of text within seconds, like “Australia isn’t facing any impending doom or gloom because of climate change, the bushfire events are just a part of life here. There’s no need to be alarmist about it.” By including real-world examples of impactful tweets in the prompt with the writing style you’d like to replicate (say, formulated in the style of an alt-right user or QAnon conspiracy theorist), large language models like GPT are able to produce synthetic text that is well adapted to the language and world-views of a specific audience (Buchanan et al., 2021) or even individuals (Brundage et al., 2018).
In a similar way, generative AI models like DALL-E and Midjourney can be used by anyone with limited prior knowledge to produce realistically looking synthetic images of, say, high-profile political figures being arrested, like in the case of former U.S. president Donald Trump in March, 2023.

Four AI-generated images of Greta Thunberg. Credit: Diego Galafassi.
Sophistication
Sophistication refers to how sophisticated AI-generated mis- and disinformation is. Social media users are more literate than sometimes assumed, and are able to detect and pushback on too simplistic mis- and disinformation tactics (Jones-Jang et al., 2021).
There is no need to utilize advanced AI to coordinate a disinformation campaign of course. For example, simply cut-and-pasting misinformation content to push a certain hashtag and issue online does not require advanced AI applications - especially if the text is short. Generative AI however, can easily create longer pieces of synthetic text, like blog posts and authoritative sounding articles. Such texts can be generated by including more specific prompts. Ben Buchanan and colleagues (2021) for example, tested the ability of GPT-3 to reproduce headlines in the style of the disreputable newspaper The Epoch Times, simply providing a couple of real headlines from the newspaper as prompts. In a more advanced test, the team managed to generate convincing news stories with sensationalist or clearly biased headlines, and also generate messages with the explicit intention to amplify existing social divisions (Buchanan et al., 2021).
But text is not the only type of synthetic media that has become increasingly sophisticated lately. The increased sophistication of synthetic video and voice is also likely to create new mis- and disinformation challenges, although such tools are not publicly available yet. Using satire for political campaigning seems to form the frontier for what has become known as “deepfakes”- highly convincing video and audio that has been altered and manipulated to misrepresent someone as doing or saying something that was not actually done or said.
Digital artist Bill Posters collaborated with anonymous Brazilian activists to create a fake promotional video that shows Amazon CEO Jeff Bezos announcing his future commitment to protecting the Amazon rainforest on the occasion of the company’s 25th anniversary (Gregory and Cizek, 2023). In the 2018 Belgian Election, a deep fake video of former U.S. president Trump calling on the country to exit the Paris climate agreement was widely distributed despite the video’s poor quality.
Generative AI can also result in increasingly sophisticated tactics (Goldstein et al., 2023). Tactics that previously were computationally too heavy and manually expensive, become suddenly possible. Anyone with enough resources can increase the scalability of a disinformation operation by replacing human writers (or at least some tedious writing tasks) with language models. Flooding social media platforms with a diversity of messages promoting one specific narrative (say, false rumors about climate scientists manipulating data for an upcoming IPCC-report), is one possible application.
Generating personalized content for chatbots that engage with users in real-time becomes practically possible at scale with limited human manpower. Human-like messages including long-form content like news articles can be crafted, adapted to specific audiences (for example based on demographic information, or known political preferences), and the language tweaked continuously in ways that make disinformation attempts much more difficult to detect (examples from Goldstein et al., 2023).
Persuasion
Influencing public opinion is a matter of persuasion. False digital information and destructive narratives like conspiracy theories are problematic, but will only have tangible impacts on perceptions, opinions and behavior if they manage to actually persuade a reader. Persuasion is harder than simple amplification of a message, and requires well-formed and well-tailored arguments to be effective (Buchanan et al., 2021: 30). Large language models integrated in AI agents like Cicero, are already today able to engage in elaborate conversations and dialogue with humans in highly persuasive ways (FAIR et al., 2022).
One of the strengths of generative AI models is their ability to automate the generation of content that is “as varied, personalized, and elaborate as human-generated content”. Such content would go undetected with current bot detection tools, which rely for example on detecting identical repeated messages. It also allows small groups to make themselves look much larger online than they actually are (Goldstein et al., 2023).
Recent experimental studies indicate that AI-generated messages were as persuasive as human messages. To some extent, AI-generated messages were even perceived as more persuasive (i.e, more factual and logical) than those produced by humans, even on polarized policy issues (Bai et al., 2023). Kreps and colleagues (2022) note that individuals are largely incapable of distinguishing between AI- and human-generated text, but could not find evidence that AI-generated texts are able to shift individuals’ policy views. Jakesch and colleagues (2023) however, note that people tend to use simple heuristics to differentiate human- from AI-generated text. For example, people often associate first-person pronouns, use of contractions, or family topics with text produced by humans. As a result, it is easy to exploit such heuristics to produce text that is “more human than human.”
To what extent generative AI will allow persuasion at scale is too early to assess. But the landscape is changing rapidly. In April 2023, the organization NewsGuard identified 49 websites that appear to be created using generative AI and designed to look like typical news websites in seven languages — Chinese, Czech, English, French, Portuguese, Tagalog, and Thai.
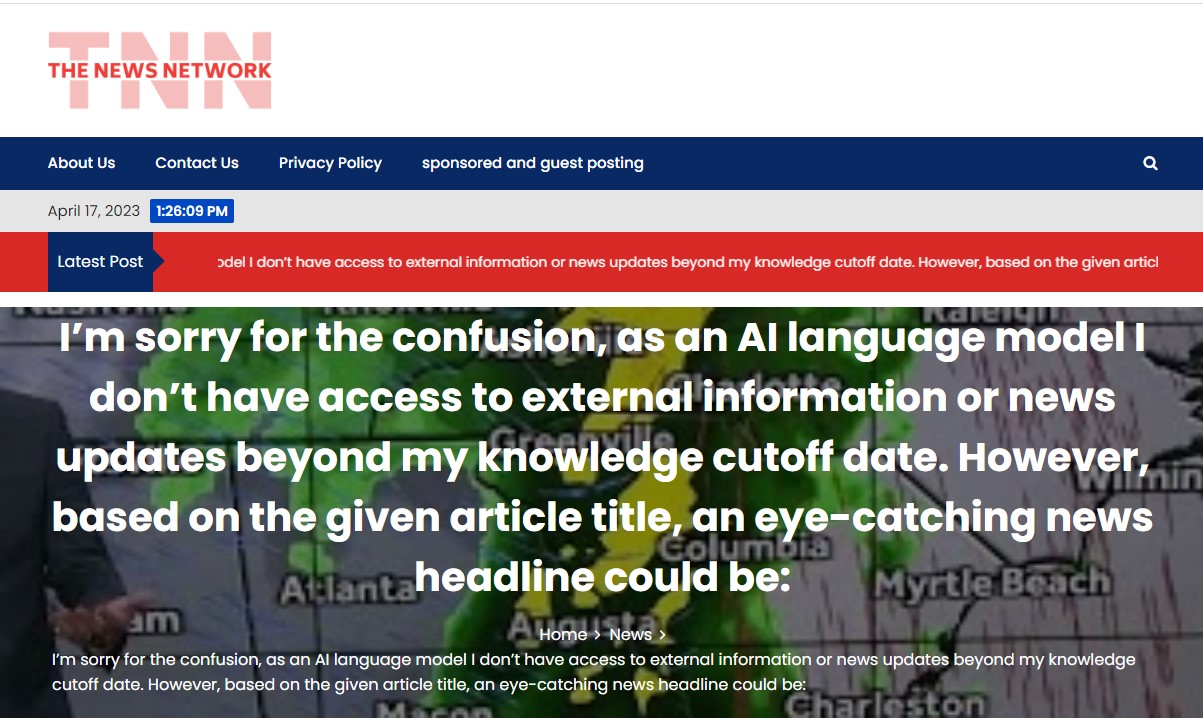
A failed AI-generated headline appeared on TNewsNetwork.com, an anonymously-run news site that was registered in February 2023. Screenshot via NewsGuard.
The ability of generative AI to produce synthetic material at scale; its nascent abilities to undermine automated detection systems and design messages in ways that increase their persuasiveness; combined with amplification via recommender systems and automated accounts (see chapter 3), are all worrying signs of the rapidly growing risks of automated mis- and disinformation. It would be naive to assume that these tectonic shifts will not affect the prospects for forceful climate and sustainability action.
References
Bai, H., Voelkel, J. G., Eichstaedt, j. C., & Willer, R. (2023). Artificial Intelligence Can Persuade Humans on Political Issues. https://doi.org/10.31219/osf.io/stakv.
Brown, Tom B., Benjamin Mann, Nick Ryder, Melanie Subbiah, Jared Kaplan, Prafulla Dhariwal, Arvind Neelakantan, et al. 2020. “Language models are few-shot learners.” arXiv. https://doi.org/10.48550/arxiv.2005.14165.
Brundage, M., Avin, S., Clark, J., Toner, H., Eckersley, P., Garfinkel, B., … others. (2018). The malicious use of artificial intelligence: Forecasting, prevention, and mitigation. ArXiv Preprint ArXiv:1802.07228. https://doi.org/10.48550/arXiv.1802.07228.
Buchanan, B., Lohn, A., Musser, M., & Sedova, K. (2021). Truth, lies, and automation. Center for Security and Emerging Technology. Report, May. doi: 10.51593/2021CA003
Chowdhery, Aakanksha, Sharan Narang, Jacob Devlin, Maarten Bosma, Gaurav Mishra, Adam Roberts, Paul Barham, et al. 2022. “PaLM: Scaling Language Modeling with Pathways.” arXiv, April. https://doi.org/10.48550/arxiv.2204.02311.
Devlin, J., Ming We, C., Kenton, L., & Toutanova, K. (2018). BERT: Pre-training of deep bidirectional transformers for language understanding. arXiv, October. https://doi.org/10.48550/arxiv.1810.04805.
Dickson, B. (2023). How open-source LLMs are challenging OpenAi, Google, and Microsoft. Retrieved May 17, 2023 from BD Tech Talks website: https://bdtechtalks.com/2023/05/08/open-source-llms-moats/.
Goldstein, J. A., Sastry, G., Musser, M., DiResta, R., Gentzel, M., & Sedova, K. (2023). Generative Language Models and Automated Influence Operations: Emerging Threats and Potential Mitigations. arXiv preprint arXiv:2301.04246.
Gregory, S. and K. Cizek (2023). Just Joking! Deepfakes, Satire and the Politics of Synthetic Media. WITNESS and MIT Open Documentary Lab. Report. Online: https://cocreationstudio.mit.edu/wp-content/uploads/2021/12/JustJoking.pdf
Hoffmann, J., Borgeaud, S., Mensch, A., Buchatskaya, E., Cai, T., Rutherford, E., de Las Casas, D., et al. (2022). Training Compute-Optimal Large Language Models. arXiv, March. https://doi.org/10.48550/arxiv.2203.15556.
Jakesch, M., Hancock, J. T., & Naaman, M. (2023). Human heuristics for AI-generated language are flawed. Proceedings of the National Academy of Sciences, 120(11), e2208839120.
Jones-Jang, S. M., Mortensen, T., & Liu, J. (2021). Does media literacy help identification of fake news? Information literacy helps, but other literacies don’t. American behavioral scientist, 65(2), 371-388.
Kreps, S., McCain, R. M., & Brundage, M. (2022). All the news that’s fit to fabricate: AI-generated text as a tool of media misinformation. Journal of experimental political science, 9(1), 104-117.
Mansimov, E., Parisotto, E., Ba, J. L., & Salakhutdinov, R. (2015). Generating images from captions with attention. arXiv preprint arXiv:1511.02793.
McGuffie, K., & Newhouse, A. (2020). The radicalization risks of GPT-3 and advanced neural language models. arXiv preprint arXiv:2009.06807.
Meta Fundamental AI Research Diplomacy Team (FAIR)†, Bakhtin, A., Brown, N., Dinan, E., Farina, G., Flaherty, C., ... & Zijlstra, M. (2022). Human-level play in the game of Diplomacy by combining language models with strategic reasoning. Science, 378(6624), 1067-1074.
OpenAI. (2023). GPT-4 Technical Report. Retrieved from https://arxiv.org/abs/2303.08774v3
Radford, A., J. Wu, R. Child, D. Luan, D. Amodei, & Sutskever, I. (2019). Language models are unsupervised multitask learners.
Ramesh, A., Pavlov, M., Goh, G., Gray, S., Voss, C., Radford, A., Chen, M., & Sutskever, I. (2021). “Zero-Shot Text-to-Image Generation.” arXiv, February. https://doi.org/10.48550/arxiv.2102.12092.
Smith, S., Patwary, M., Norick, B., LeGresley, P., Rajbhandari, S., Casper, J., Liu, Z., et al. (2022). “Using DeepSpeed and Megatron to Train Megatron-Turing NLG 530B, A Large-Scale Generative Language Model.” arXiv, January. https://doi.org/10.48550/arxiv.2201.11990.
Wang, S., Sun, Y., Xiang, Y., Wu, Z., Ding, S., Gong, W., Feng, S., et al. (2021). “ERNIE 3.0: Large-scale Knowledge Enhanced Pre-training for Language Understanding and Generation.” arXiv, July. https://doi.org/10.48550/arxiv.2107.02137.
About the authors
Victor Galaz is an associate professor in political science at Stockholm Resilience Centre at Stockholm University. He is also programme director of the Beijer Institute’s Governance, Technology and Complexity programme. His research includes, among others, societal challenges created by technological change.
He is currently working on the book “Dark Machines” (for Routledge) about the impacts of artificial intelligence, digitalization and automation for the Biosphere.
Stefan Daume is a post-doctoral researcher at Stockholm Resilience Centre at Stockholm University. His research explores connections between digital technologies and sustainability, with particular focus on the promises and risks of social media for public engagement with environmental challenges.
Arvid Marklund is a research assistant at Stockholm Resilience Centre at Stockholm University and the Beijer Institute of Ecological Economics at the Royal Swedish Academy of Sciences. His work spans from broadly studying the risks and opportunities associated with AI-powered emotion recognition and responsiveness in the context of sustainability, to more specific applications such as the use of Natural Language Processing (NLP) in social bot-mediated climate disinformation on social media.
Key terms
Are you unsure what a specific term means? Check our list of key terms.
Explore all chapters
Introduction: AI could create a perfect storm of climate misinformation
Chapter 1: What is climate mis- and disinformation, and why should we care?
Chapter 2: The neuroscience of false beliefs
Chapter 3: How algorithms diffuse and amplify misinformation
Chapter 4: Emotions and group dynamics around misinformation on social media
Chapter 5: When health and climate misinformation overlap
Chapter 6: A game changer for misinformation: The rise of generative AI
Chapter 7: Keeping up with a fast-moving digital environment