Chapter 4
Emotions and group dynamics around misinformation on social media
Lead author: Hannah Metzler, Complexity Science Hub Vienna
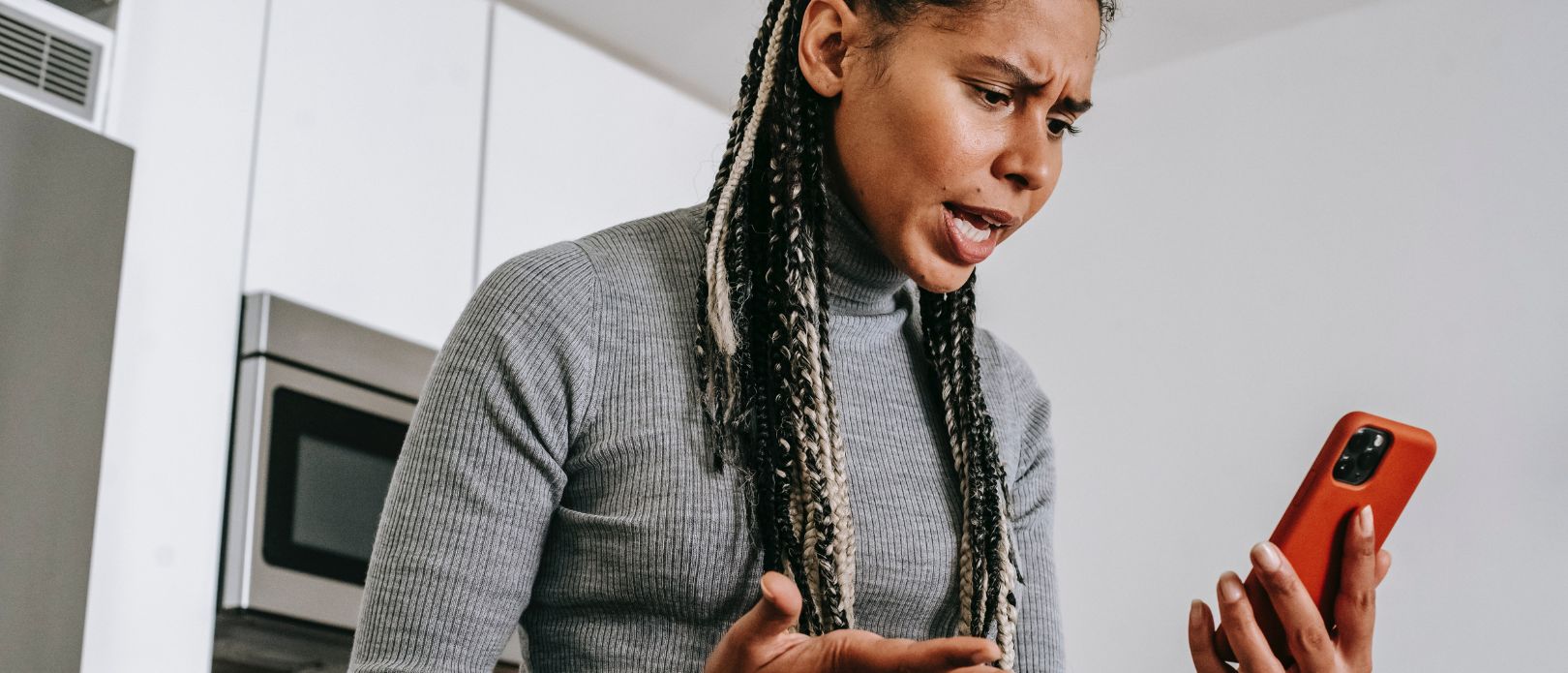
Extreme voices and toxic content are more visible on social media. Photo: Alex Green via Pexels.
Nuanced views and clean facts don’t generate clicks. Social networks are designed to speak to our emotions; the more extreme the emotions, the better the content. But that does not mean that good arguments, education, and science communication are futile.
Emotions attract our attention, and provide us with information about actions we should take: When you feel fear, it’s best not to ignore the danger, and protect yourself from it. When you are angry, it’s probably because someone has treated you or a group you belong to unfairly, and it’s time to step up against the injustice.
News agencies, politicians, and creators of fake news know this, and use it to create content that attracts attention and is likely to be shared on digital and social media. Algorithms on social media are optimized to increase engagement with content (Narayanan, 2023; Metzler & Garcia, 2022), and content that provokes strong emotional reactions is a powerful means to do so. Negative moral-emotional messages about groups we do not like seem to particularly increase engagement (Brady et al., 2017, Rathje et al. 2021, Antoine et al., 2023). Humans are social animals, and things that make us feel part of a group, that increase our group’s status, or decrease the status of an out-group, are highly motivating for us (Robertson et al., 2022).
We can regularly observe such emotional group dynamics around the topic of climate change on social media. On the one hand, there are people who think we are not doing enough and need to urgently take action: social movements like Fridays for Future or Extinction Rebellion, and political parties like the Green parties in Europe, or Democrats in the US. On the other side, climate scepticism and denial are more common in far-right, populist or libertarian parties, who oppose economic regulation and benefit from using anti-elite rhetoric (Lewandowsky, 2021). Polarized conversations between these different sides on social media grow around events like climate protests, or releases of climate change reports, such as the reports from the Intergovernmental Panel on Climate Change, (Sanford, 2021). And political polarization fuels the spread of misinformation (Osmundsen et al., 2021, Antoine et al., 2023).
Because more outrageous content attracts more attention, and individuals with stronger opinions are more motivated to persuade others, extreme voices and toxic content are more visible on social media (Bail, 2021). Individuals with more nuanced views, who can relate to both sides of a debate, are much less visible. A large majority of individuals who are not interested enough to participate in discussions, but generally agree with a nuanced perspective, is entirely invisible. This way, digital media make polarization seem stronger than it actually is in society, and this in turn fuels hate and misunderstanding between parties (Brady et al. 2023). Redesigning platforms so that nuanced majorities and overlap in the views of different groups become more visible, could therefore help to decrease the spreading of misinformation (Metzler & Garcia, 2022). Redesigning social media algorithms could be one way to do so.
Fortunately, people do not uncritically believe any emotional information that comes their way (Mercier, 2020). Key questions such as who shares news, whether they know and trust the source, and if it fits with what they already know and believe about the world, crucially determine if we find information plausible. People's anger after reading false news, for example, can occur because they recognize it as misinformation and disagree (Lühring et al., 2023). So, strong emotions do not automatically mean people will believe a message and continue to share it. That people judge new information based on trust in sources, and their knowledge about the world, means that good arguments, education, and science communication are not futile. Explaining how we know climate change is happening, how it works, how solutions can integrate economic and environmental needs, for example, takes time and effort. But it will also help to increase trust in science, and politics that implements such evidence-based solutions, and thereby decrease polarization and misinformation.
References
Bail, C. A. (2021). Breaking the social media prism: How to make our platforms less polarizing. Princeton University Press.
Brady, W. J., McLoughlin, K. L., Torres, M. P., Luo, K. F., Gendron, M., & Crockett, M. J. (2023). Overperception of moral outrage in online social networks inflates beliefs about intergroup hostility. Nature Human Behaviour, 1–11. https://doi.org/10.1038/s41562-023-01582-0
Brady, W. J., Wills, J. A., Jost, J. T., Tucker, J. A., & Bavel, J. J. V. (2017). Emotion shapes the diffusion of moralized content in social networks. Proceedings of the National Academy of Sciences, 114(28), 7313–7318. https://doi.org/10.1073/pnas.1618923114
Lewandowsky, S. (2021). Climate Change Disinformation and How to Combat It. Annual Review of Public Health, 42(1), 1–21. https://doi.org/10.1146/annurev-publhealth-090419-102409
Lühring, J., Shetty, A., Koschmieder, C., Garcia, D., Waldherr, A., & Metzler, H. (2023). Emotions in misinformation studies: Distinguishing affective state from emotional response and misinformation recognition from acceptance. PsyArXiv. https://doi.org/10.31234/osf.io/udqms
Marie, A., Altay, S., & Strickland, B. (2023). Moralization and extremism robustly amplify myside sharing. PNAS Nexus, 2(4), pgad078. https://doi.org/10.1093/pnasnexus/pgad078
Mercier, H. (2020). Not Born Yesterday. https://press.princeton.edu/books/hardcover/9780691178707/not-born-yesterday
Metzler, H., & Garcia, D. (2022). Social drivers and algorithmic mechanisms on digital media. PsyArXiv. https://doi.org/10.31234/osf.io/cxa9u
Narayanan, A. (2023). Understanding Social Media Recommendation Algorithms. Knight First Amend. Inst., 23(01). https://perma.cc/F3NP-FEQX
Osmundsen, M., Bor, A., Vahlstrup, P. B., Bechmann, A., & Petersen, M. B. (2021a). Partisan Polarization Is the Primary Psychological Motivation behind Political Fake News Sharing on Twitter. American Political Science Review, 115(3), 999–1015. https://doi.org/10.1017/S0003055421000290
Osmundsen, M., Bor, A., Vahlstrup, P. B., Bechmann, A., & Petersen, M. B. (2021b). Partisan Polarization Is the Primary Psychological Motivation behind Political Fake News Sharing on Twitter. American Political Science Review, 115(3), 999–1015. https://doi.org/10.1017/S0003055421000290
Rathje, S., Bavel, J. J. V., & Linden, S. van der. (2021). Out-group animosity drives engagement on social media. Proceedings of the National Academy of Sciences, 118(26). https://doi.org/10.1073/pnas.2024292118
Robertson, C. E., Pretus, C., Rathje, S., Harris, E., & Van Bavel, J. J. (2022). How Social Identity Shapes Conspiratorial Belief. Current Opinion in Psychology, 101423. https://doi.org/10.1016/j.copsyc.2022.101423
Sanford, M., Painter, J., Yasseri, T., & Lorimer, J. (2021). Controversy around climate change reports: A case study of Twitter responses to the 2019 IPCC report on land. Climatic Change, 167(3), 59. https://doi.org/10.1007/s10584-021-03182-1
About the author
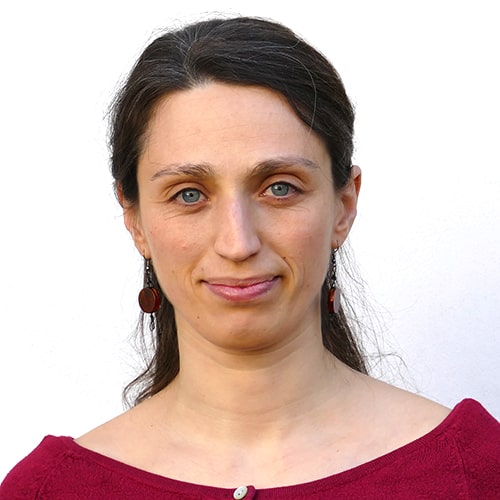
Hannah Metzler is a postdoctoral researcher at the Complexity Science Hub Vienna. Her main research interest lies in the role of emotions and social processes in communication.
In her current research, she applies methods like text analysis and machine learning to capture emotions at the collective level, and investigate their effects on misinformation spreading on social media.
Key terms
Are you unsure what a specific term means? Check our list of key terms.
Explore all chapters
Introduction: AI could create a perfect storm of climate misinformation
Chapter 1: What is climate mis- and disinformation, and why should we care?
Chapter 2: The neuroscience of false beliefs
Chapter 3: How algorithms diffuse and amplify misinformation
Chapter 4: Emotions and group dynamics around misinformation on social media
Chapter 5: When health and climate misinformation overlap
Chapter 6: A game changer for misinformation: The rise of generative AI
Chapter 7: Keeping up with a fast-moving digital environment